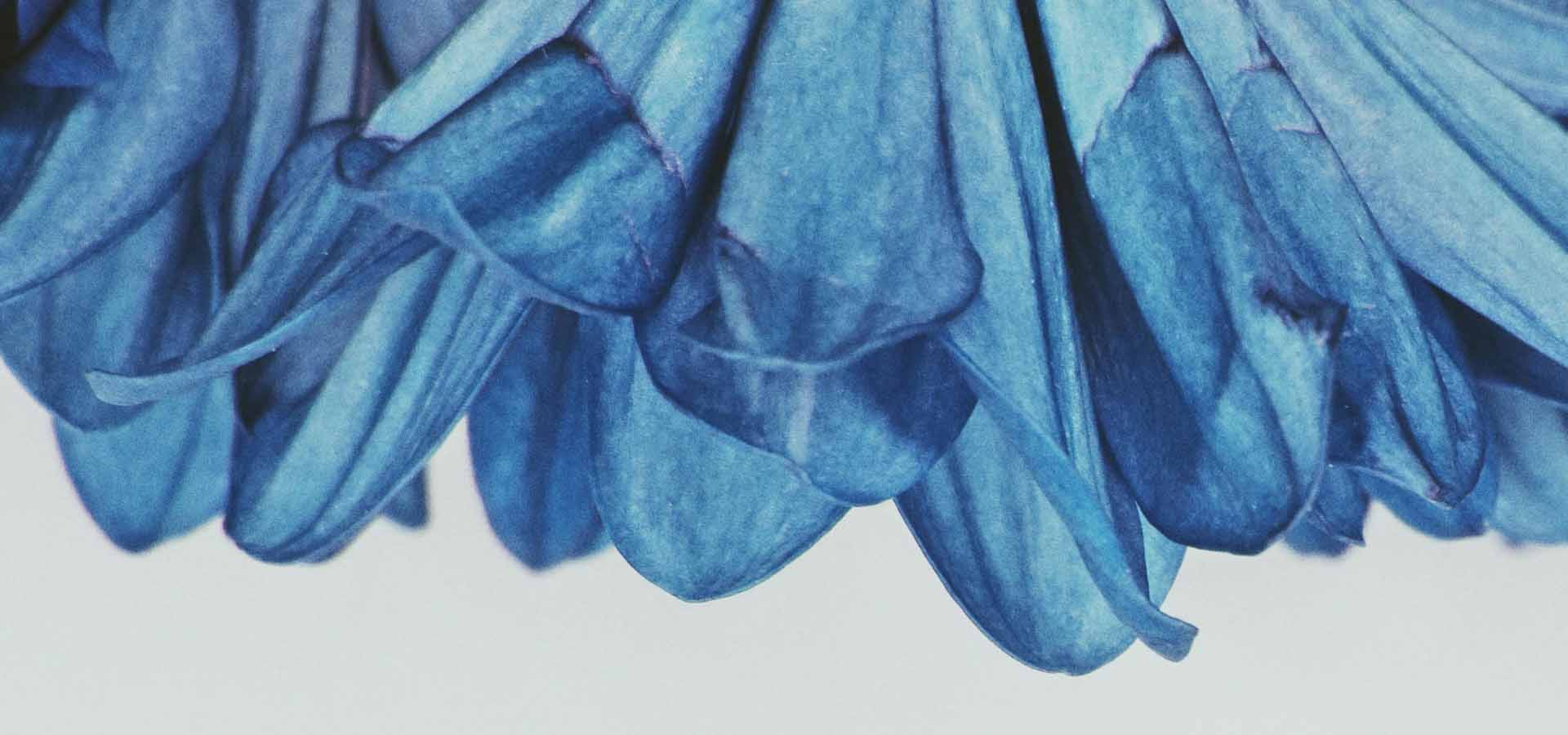
Molecular profiling and drug prediction in ovarian cancer patients
Research Project | 01.04.2022 - 31.03.2026
Personalized treatment in oncology requires predictive biomarkers and direct on-target drug response to tailor therapy regimens. However, many efforts to develop truly predictive biomarkers are limited due to only a handful of genetic aberrations demonstrating clear clinical benefit for ‘targeted’ treatments. Together with an emerging trend in using drug combinations rather than single compounds, large prospective clinical trials require long time and enormous investments to demonstrate only marginal benefits. In order to overcome these limitations hampering major breakthroughs, researchers have moved towards ex vivo screening or patient-derived xenograft models envisioning to provide clinically relevant data in a 4-week turnaround. Here we aim to combine single-cell transcriptomics with drug treatment on ex vivo spheroids in a prospectively collected and homogenous cohort of ascites samples derived from high-grade serous ovarian cancer patients to 1) define the cell composition of ascites, an abdominal fluid with malignant cells taken through regular drainage to release patients’ pain; 2) align patients outcome with molecular and cellular signatures and ex vivo drug response, 3) evaluate in silico predicted and cancer cell-specific drug response in patient-derived cultures, and 4) ultimately define novel non-genetic biomarker-drug axes improving personalized treatment. In detail, we will test currently applied and suggested drug regimens in real-time using a straightforward ex vivo spheroid culture system. With an already established single-cell RNA sequencing pipeline we will also identify individual patient tumor heterogeneity and evaluate in silico drug response ex vivo. Our ex vivo spheroid platform will test cell proliferation, apoptosis, and response to single drugs and combinations in ascites-derived samples. Our bimodal approach is novel and the consideration of tumor heterogeneity together with drug response in patients’ material has not been addressed. Thus, we believe that the setup described in this grant can be further developed into a clinical helpful predictive analysis scheme. Moreover, predictors and response patterns delivered by this proposal will be useful for their testing in future ovarian cancer clinical trials.