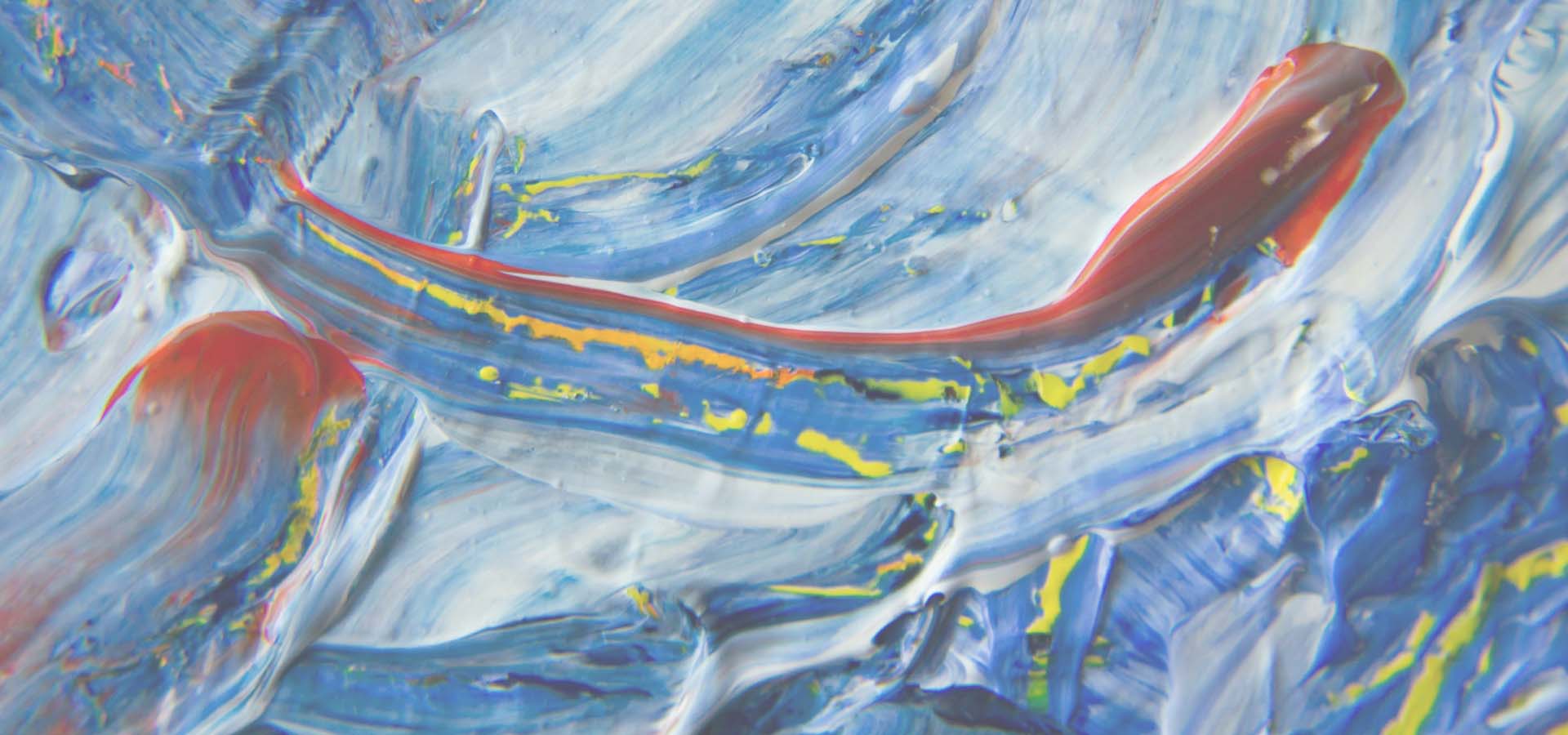
Machine learning techniques for personalized breast cancer prognosis - Swiss BCpro
Research Project | 01.06.2021 - 31.05.2022
Early detection of breast cancer through screening and the advent of new treatments has contributed greatly to disease survival. This, in turn, elevates the importance of disease prognosis i.e., survival and cancer recurrence. A prognostic model with increased ability to classify patients into different risk groups and estimate overall survival time can influence choice of treatment and can spare patients from unnecessary treatments. Clinicians can offer patients individualized treatment and disease management strategies if a reliable and accurate prognostic model is available and has been incorporated in clinical practice. Most patients also desire information about their prognosis and overall survival. Researchers can design more efficient and ethical clinical trials by using accurate prognostic models to stratify patients. Finally, prognostic models have a great impact on health policy and allocation of social resources among cancer survivors. In the past two decades, several models have been developed for breast cancer prognosis.
Most models have been developed to test the prognostic value of a specific biomarker or to compare methods for model development, rather than to support clinical decision-making. Few models went through the complete translational pathway from preclinical development, validation, to the evaluation of clinical usefulness, such as the improvement of clinical decision-making. Only one model, which can classify patients into two risk groups (Dying vs. Surviving) was tested for "clinical usefulness".
Classifying patients into two groups cannot reflect its usefulness in clinical settings, since there are numerous treatments, treatment decisions are based on various factors and often change over time. A clinically useful prognostic model should be able to classify patients into multiple risk groups so that it can have mutual corroboration with treatment options and guidelines. The model eventually needs to be accessed in a randomized control trial, testing whether using the model to classify patients into different risk groups and informing treatment decisions improves survival or other patient reported outcomes.
Finally, the performance of current models varies across different populations. No model was developed with patients from Switzerland, validated for the Swiss population, or has been evaluated for clinical usefulness in Swiss clinical settings. Machine learning offers an alternative approach to classical model-based prediction e.g. Cox proportional hazard (PH) regression. It can address limitations of classical modeling and improve accuracy from 70% of Cox PH models to about 85%. A machine learning-based prognostic model learns from real world data where more than 20% breast cancer treatment decisions disagree with clinical guidelines and change over time. It can classify breast cancer patients into multiple risk groups, and also generate risk predictions based on different treatments.
The treatment-specific stratification can inform treatment decision-making. However, no machine learning-based breast cancer prognostic model has been validated in independent populations for generalizability, and no machine learning-based model was tested for clinical usefulness, such as supporting clinical decision-making. We aim to create a machine learning-based breast cancer prognostic model, the Swiss-BCpro, to support personalized management of breast cancer with data collected from Swiss cancer registries.
The specific aims are 1. to develop the Swiss-BCpro by using machine learning to analyze data available in the Geneva cancer registry; 2. to compare the calibration and predictive accuracy of Swiss-BCpro with other state-of-the-art models for breast cancer prognosis i.e., PREDICT, NPI, CancerMath; 3. to externally validate Swiss-BCpro with independent data from the cancer registries of Zurich and St. Gallen; 4. to explore its clinical usefulness, namely comparing the clinical utility of Swiss-BCpro stratification with both guideline-based clinical decision-making and actual treatments patients received.
The study will contribute to the personalized management of breast cancer patients in Switzerland by translating machine leaning-based breast cancer prognostic modeling into clinical practice.
Follow this link for further information