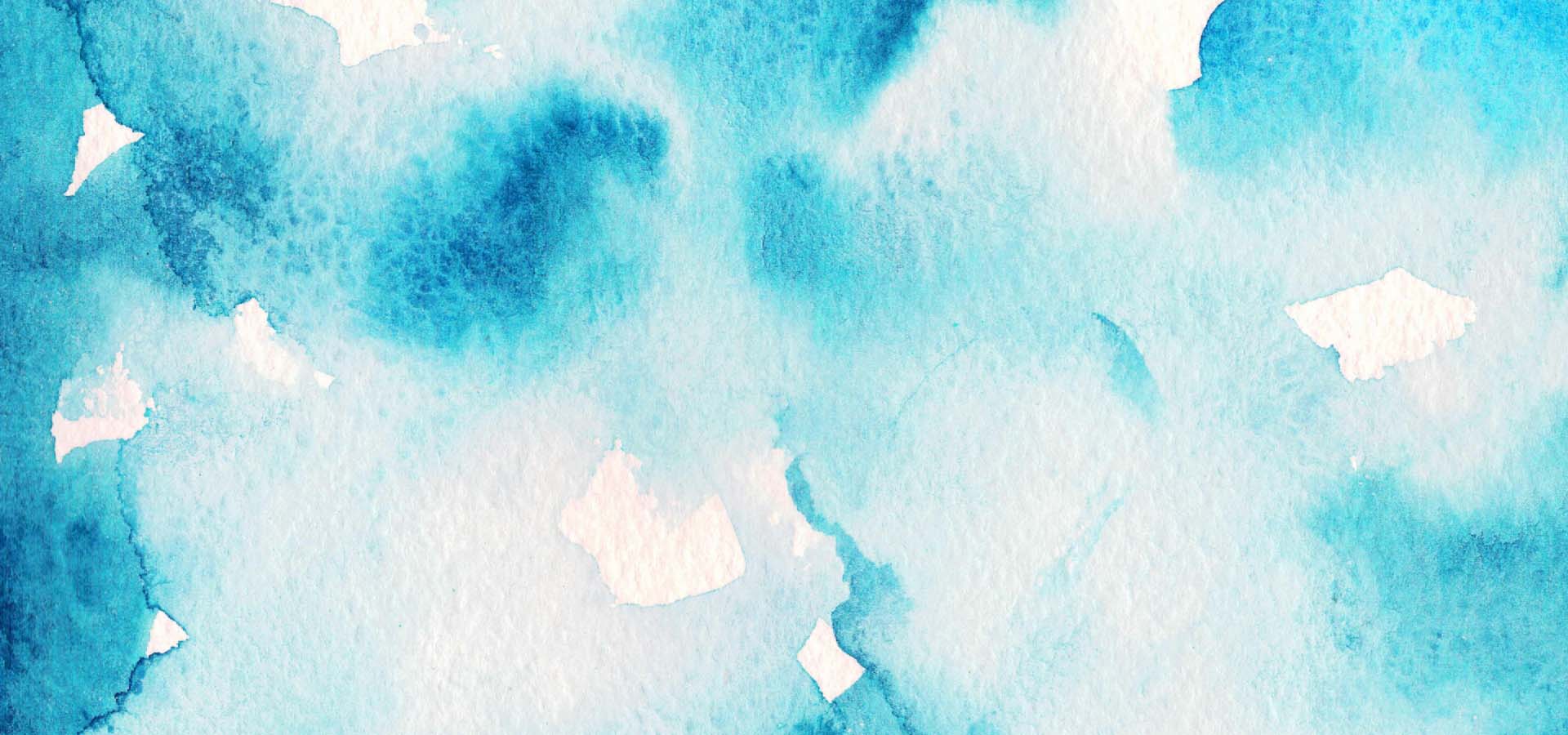
Causality and Physics-informed Machine Learning
Research Project | 01.01.2017 - 31.12.2025
|
01.01.2017
- 31.12.2025
Publications
Nagy-Huber, Monika and Roth, Volker (2024) ‘Physics-informed boundary integral networks (PIBI-Nets): A data-driven approach for solving partial differential equations’, Journal of Computational Science, 81. Available at: https://doi.org/10.1016/j.jocs.2024.102355.
Nagy-Huber, Monika and Roth, Volker (2024) ‘Physics-informed boundary integral networks (PIBI-Nets): A data-driven approach for solving partial differential equations’, Journal of Computational Science, 81. Available at: https://doi.org/10.1016/j.jocs.2024.102355.
Arend Torres, Fabricio et al. (2024) ‘Lagrangian Flow Networks for Conservation Laws’, in The Twelfth International Conference on Learning Representations. Vienna, Austria (The Twelfth International Conference on Learning Representations). Available at: https://openreview.net/forum?id=Nshk5YpdWE.
Arend Torres, Fabricio et al. (2024) ‘Lagrangian Flow Networks for Conservation Laws’, in The Twelfth International Conference on Learning Representations. Vienna, Austria (The Twelfth International Conference on Learning Representations). Available at: https://openreview.net/forum?id=Nshk5YpdWE.
Negri, Marcello Massimo, Arend Torres, Fabricio and Roth, Volker (2023) ‘Conditional Matrix Flows for Gaussian Graphical Models’, in Advances in Neural Information Processing Systems. New Orleans: Curran Associates, Inc. (Advances in Neural Information Processing Systems), pp. 25095––25111. Available at: https://proceedings.neurips.cc/paper_files/paper/2023/file/4eef8829319316d0b552328715c836c3-Paper-Conference.pdf.
Negri, Marcello Massimo, Arend Torres, Fabricio and Roth, Volker (2023) ‘Conditional Matrix Flows for Gaussian Graphical Models’, in Advances in Neural Information Processing Systems. New Orleans: Curran Associates, Inc. (Advances in Neural Information Processing Systems), pp. 25095––25111. Available at: https://proceedings.neurips.cc/paper_files/paper/2023/file/4eef8829319316d0b552328715c836c3-Paper-Conference.pdf.
Arend Torres, Fabricio et al. (2022) ‘Mesh-free eulerian physics-informed neural networks’. Available at: https://doi.org/10.48550/arxiv.2206.01545.
Arend Torres, Fabricio et al. (2022) ‘Mesh-free eulerian physics-informed neural networks’. Available at: https://doi.org/10.48550/arxiv.2206.01545.
Parbhoo, Sonali et al. (2020) ‘Information Bottleneck for Estimating Treatment Effects with Systematically Missing Covariates’, Entropy, 22(4), p. 389. Available at: https://doi.org/10.3390/e22040389.
Parbhoo, Sonali et al. (2020) ‘Information Bottleneck for Estimating Treatment Effects with Systematically Missing Covariates’, Entropy, 22(4), p. 389. Available at: https://doi.org/10.3390/e22040389.
Wieczorek, Aleksander and Roth, Volker (2020) ‘On the Difference between the Information Bottleneck and the Deep Information Bottleneck’, Entropy, 22(2), p. 131. Available at: https://doi.org/10.3390/e22020131.
Wieczorek, Aleksander and Roth, Volker (2020) ‘On the Difference between the Information Bottleneck and the Deep Information Bottleneck’, Entropy, 22(2), p. 131. Available at: https://doi.org/10.3390/e22020131.
Wieser, Mario et al. (2020) ‘Inverse Learning of Symmetries’, in Larochelle, H.; Ranzato, M.; Hadsell, R.; Balcan, M. F.; Lin, H. (ed.). Curran Associates, Inc.: Curran Associates, Inc.
Wieser, Mario et al. (2020) ‘Inverse Learning of Symmetries’, in Larochelle, H.; Ranzato, M.; Hadsell, R.; Balcan, M. F.; Lin, H. (ed.). Curran Associates, Inc.: Curran Associates, Inc.
Wieczorek, Aleksander and Roth, Volker (2019) ‘Information Theoretic Causal Effect Quantification’, Entropy, 21(10), p. 975. Available at: https://doi.org/10.3390/e21100975.
Wieczorek, Aleksander and Roth, Volker (2019) ‘Information Theoretic Causal Effect Quantification’, Entropy, 21(10), p. 975. Available at: https://doi.org/10.3390/e21100975.
Wieczorek, Aleksander et al. (2018) ‘Learning sparse latent representations with the deep copula information bottleneck’. International Conference on Learning Representations, ICLR.
Wieczorek, Aleksander et al. (2018) ‘Learning sparse latent representations with the deep copula information bottleneck’. International Conference on Learning Representations, ICLR.