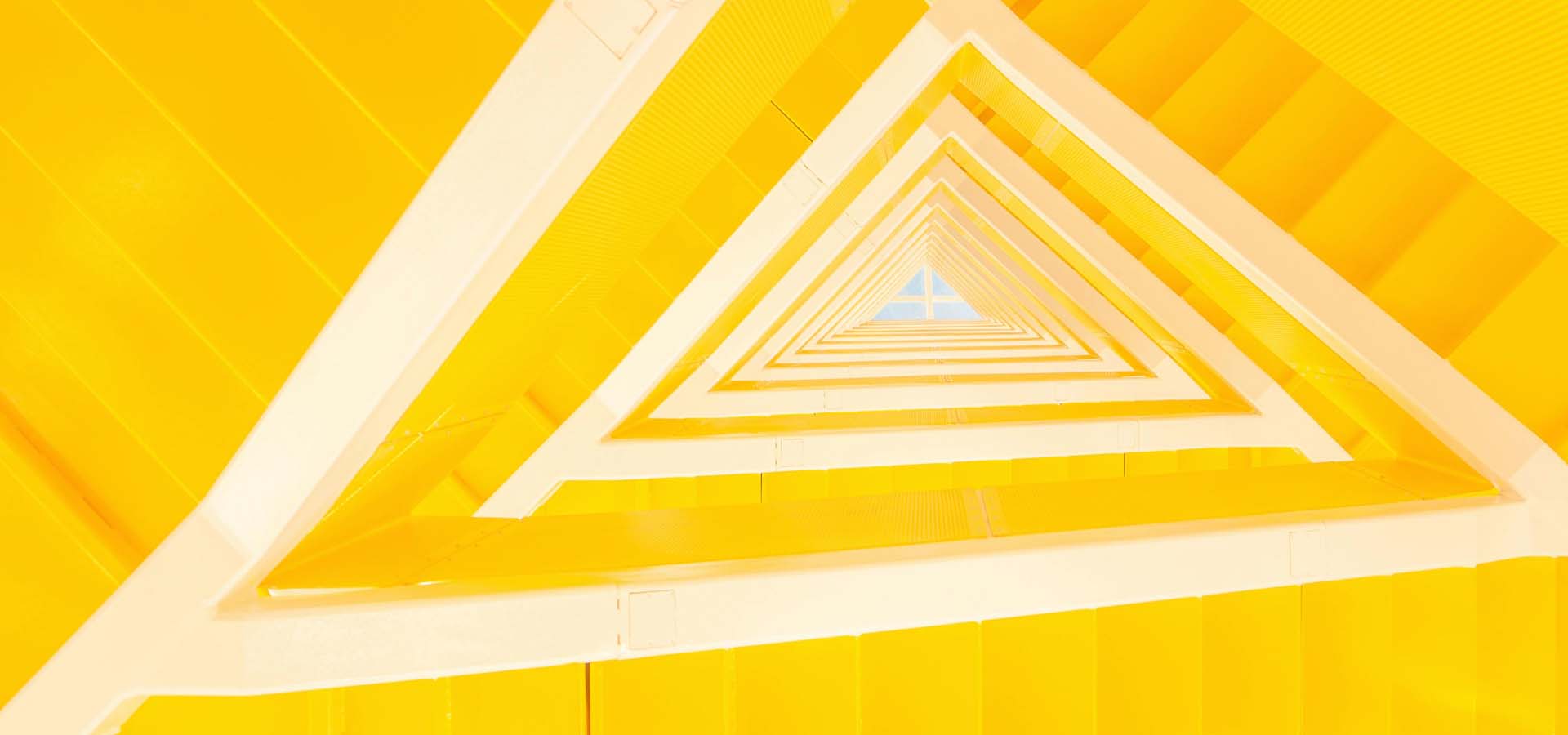
MARVEL Machine Learning Incubator
Research Project | 01.01.2018 - 31.05.2023
|
01.01.2018
- 31.05.2023
Publications
Nesterov, Vitali et al. (2022) ‘Learning Invariances with Generalised Input-Convex Neural Networks’. Available at: https://doi.org/10.48550/arxiv.2204.07009.
Nesterov, Vitali et al. (2022) ‘Learning Invariances with Generalised Input-Convex Neural Networks’. Available at: https://doi.org/10.48550/arxiv.2204.07009.
Nesterov, Vitali, Wieser, Mario and Roth, Volker (2020) ‘3DMolNet: A Generative Network for Molecular Structures’, Arxiv [Preprint]. Cornell University (Arxiv). Available at: https://doi.org/10.48550/arxiv.2010.06477.
Nesterov, Vitali, Wieser, Mario and Roth, Volker (2020) ‘3DMolNet: A Generative Network for Molecular Structures’, Arxiv [Preprint]. Cornell University (Arxiv). Available at: https://doi.org/10.48550/arxiv.2010.06477.
Wieser, Mario et al. (2018) ‘Learning Sparse Latent Representations with the Deep Copula Information Bottleneck’. ICLR: ICLR. Available at: https://openreview.net/forum?id=Hk0wHx-RW.
Wieser, Mario et al. (2018) ‘Learning Sparse Latent Representations with the Deep Copula Information Bottleneck’. ICLR: ICLR. Available at: https://openreview.net/forum?id=Hk0wHx-RW.